Cut Through the Data Craze With Collaborative Analytics
When business analytics first became available to US companies in the 1950s, capabilities were limited and technology was often prohibitively expensive. It was decades before most companies made analytics a standard practice in their organizations. Business leaders continued to rely on their experience and intuition to make critical decisions. But as analytics technology evolved, insights became more valuable and leaders took notice. By the 1980s, most large companies were employing some form of analytics for decision support.
Fast forward 30 years and companies like General Electric are making multi-billion dollar investments in their analytics infrastructures. It’s more than just Big Data; leaders know that their data is only as valuable as the insights it provides. As analytics have evolved and improved, decision-makers are demanding more from their analytics than ever.
Supply chain leaders specifically are no longer content with ad hoc systems that leave the door open for human error and bias. They know they will never hit aggressive inventory and delivery goals if their team members work alone on “islands” with inefficient processes and backward-looking insights.
The future of supply chain analytics is predictive, prescriptive, and collaborative.
This post will explore the evolution of supply chain analytics and shed light on what lies in store for manufacturers as they implement more effective tools in the future. Does your supply chain employ backward-looking analytics that only provide historical data? Or does your system provide high level information about where your organization has been and what steps you should take for improvement? Is your company’s approach more focused on the data or the people using it? Keep these questions in mind as we take a look at the evolution of analytics and how new approaches can lead to increased performance and profitability.
The Old Guard: Descriptive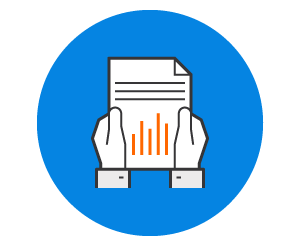
“What was the outcome?”
For the first 50 years of use, modern business analytics were descriptive, using graphs and charts to provide summaries of historical data. Slow, backward-looking, and menial, statistical analysis often required weeks or months to complete. For the first few decades, leaders did not make decisions based directly on analytics. In fact, most data teams were far-removed from the decision-making process altogether. But as insights proved more valuable, executives began relying on them more heavily.
Even after their processes were digitized, data teams continued to spend the bulk of their work hours gathering and preparing data for analysis. In addition to the sizeable manual workload, there was a lack of standardized processes, and nothing in the way of insights about the future. Supply chains were simply not getting the most out of their analytics.
A descriptive approach was adequate for small data sources in a time when most companies treated analytics as marginal to their strategies and everyday decisions. Backward-looking statistics had many limitations. Complex macros executed in spreadsheets provided no projections for future outcomes, which left decision-makers relying on experience and intuition, rather than accurate scientific models. No explanations were provided about root causes of inventory issues so decisions were made by guessing at how to drive improvement.
As the decades went on, manufacturers advanced in almost every area, from the factory floor to the boardroom. But throughout all the growth and advancement between the 1950s and 1990s, the approach to supply chain analytics remained largely static. Analysts in “back rooms” were expected to spend much more time getting data ready for analysis than actually performing analyses. Executives continued to make critical decisions based on static, backward-looking data and their best guesses as to what would happen next. It was time for better ways.
The New Guard: Predictive
“What is the future outcome?”
During the 1990s, ERP systems became ubiquitous. Most large companies had one installed and running by the year 2000. This opened up new worlds of possibility for analytics, but manufacturers were limited because analytics software and approaches were not evolved enough to handle the large data sets that were now available. In the mid-2000s, Business Intelligence (BI) software became more common in the supply chain space. Instead of only backward-looking descriptive reports, BI tools provided supply chain decision-makers with projections of future outcomes based on past trends.
For the first time, manufacturers were able to take advantage of technological advancements that made analytics insights more accessible to decision makers. Mass amounts of data could now be stored at scale. Real-time processing brought deep system data, allowing for quicker and easier manipulation. More agile, forward-looking analytics gave companies a competitive advantage. Predictive analytics had arrived, and had brought a new era with it.
Companies like SAP, SAS, and IBM were quick to develop forward-looking software that provided manufacturers with far more valuable and actionable insights than descriptive approaches. Leaders now had access to graphs and charts that projected future outcomes based on historical data. Using these insights, they could develop strategies and make adjustments to improve future outcomes.
While the advancement from descriptive to predictive has indeed been revolutionary, many challenges have remained. BI tools require highly-skilled analysts to spend large chunks of their workdays collecting and entering data for analysis. Most BI tools are not purpose-built for manufacturing, which means supply chains have to pay for costly custom development build-outs on the front end. And predictions are only based on past trends, which continues to leave decision-makers in the dark about how to make improvements. These and many more challenges continue to hinder supply chain professionals as they rely on outdated analytics models and processes.
The Upstart: Prescriptive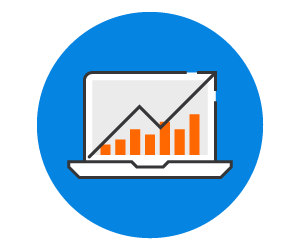
“What is the best possible outcome?”
The advent of artificial intelligence (AI) has driven a seismic shift in how supply chains analyze their data and the goals they pursue. With so much data available, companies can no longer rely on BI tools which require specialized skills, too much development time, and leave decision-makers guessing. Supply chains of the future are relying on AI and machine learning to guide their teams to make better decisions and create better workflows.
Newer, AI-backed software solutions offer a prescriptive approach. Future predictions are combined with suggested actions that result in the best possible outcomes. Rather than spending hours gathering and preparing data, teams take advantage of automated collection and analysis, which frees up their brain power to focus on working strategically. With a single click, analysts, buyers, managers, and executives can see historical trends, future projections, and recommended next steps for improving outcomes.
Prescriptive analytics simplifies and automates decisions as your teams work to reduce and optimize inventory, improve on-time delivery, and maximize profitability. Competitive, forward-looking supply chains are using prescriptive software to save their teams untold hours on manual work and human error. And while prescriptive analytics may sound like the future, a new horizon is already in view.
The Visionary: Collaborative
“How do we work together to deliver the best outcome?”
Predictive and prescriptive analytics are powerful tools, but they are only one piece of a larger puzzle. Working in a “bubble,” each with their own reports and methods, leaves users disconnected from their team’s common goals and KPIs. Workflows are not standardized, which means team members rely on tribal knowledge to complete tasks and have no practical method for sharing best practices. Each person works on their own little island, in many ways cut off from the rest of their team.
Collaborative analytics represent the next evolution for supply chains. It combines the automated decision-making of predictive and prescriptive analytics with real-time collaboration capabilities in a simple, cloud-based software that brings together buyers, suppliers, and leadership to solve challenges and drive improvement. Leaders responsible for inventory and their teams share information about everything from best practices to procurement strategy to supplier performance and much more.
In a collaborative analytics platform, up-to-date information is available from any device in the world, including a history of actions and communiques across all teams. It’s about far more than dashboards and reports; teams at separate sites are unified behind common goals. They communicate and share information in real time to optimize inventory, avoid costly disruptions, and improve both supplier and customer delivery performance.
For example, buyers at one site can alleviate shortages by purchasing inventory at cost from another. Supplier performance initiatives are assigned and tracked to completion, with automated scorecards available to every stakeholder in real time. And executives globally have access to the same information at the same time. They can see high and low performers as well as track assigned actions being taken to achieve the company’s strategic goals.
Technologies collide where real-time collaboration integrates with big data analytics and AI-based algorithms. The breakout of data manipulation and report delivery, decision-making, and actioning ends when all three come together in one platform. Teams work together to solve problems identified by the data and see it through to implementation, transforming the way they deliver value to their organization.
Conclusion
“Bring People Together”
Think about your supply chain’s approach to procurement, analytics, reporting, and overall decision-making. Are they agile enough to allow you to think beyond data and see the big picture? Do your tools bring people together to solve problems in a strategic way? Do they give you the information you need, when you need it, to make the most profitable decisions to hit aggressive goals? Can your stakeholders work as a team to predict and manipulate future outcomes? Is your supply chain more focused on the people who deliver real value to the company than data? If you answered no to these questions, it’s a good time to develop new approaches to old work.
AI is transforming how analytics deliver value to manufacturers, but it alone is not enough to transform your supply chain. It’s the people in your organization who will drive innovation, improve profitability, and deliver savings. In order to see real change, you must provide your teams with the most effective tools in a platform that guides them to work together to achieve common goals. And you must relieve them of the long, manual processes that keep their brain power tied up. When they can work strategically and collaboratively, your people will deliver the transformation.
LeanDNA brings your talent together into a cutting-edge collaborative tool. Manufacturers around the world depend on LeanDNA to end firefighting mode, keep inventory levels reasonable, and improve on-time delivery performance by bringing their people together behind strategic goals. When teams work together, supply chains are transformed.
Learn more about how LeanDNA can help you reach your goals through collaborative analytics by watching our two minute video, LeanDNA Explained, or, just give us a call and we’ll share what we have learned through years of working with manufacturing customers just like you.
Ready for more? Check out the associated infographic here.
Looking for the best way to unite people?
Siloed data is one of the main drivers of confusion in supply chain operations. Unified teams make better supply chain decisions. New analytics technology is aiding teams in creating better supply chain collaboration that deals with data siloes and connects teams in a way that increases efficiency and optimizes procurement processes.
Learn more about the trouble with one-way analytics.
Today supply chain is more global, and sychronization is becoming increasingly vital.
This includes not only the synchronization of teams, but the sychronization of suppliers throughout the supply chain as well. When organizations need to quickly meet changing demands, and pressures to deliver on time regardless of the product complexity and routine demand changes, they are more likely to complete tasks in a connected supply chain.
Learn how how collaborative analytics empowers agile supply chain procurement.